Archaic Coastal Settlement in Eastern North America
Tracing Native American Mound Building Practices Across the Southeastern United States
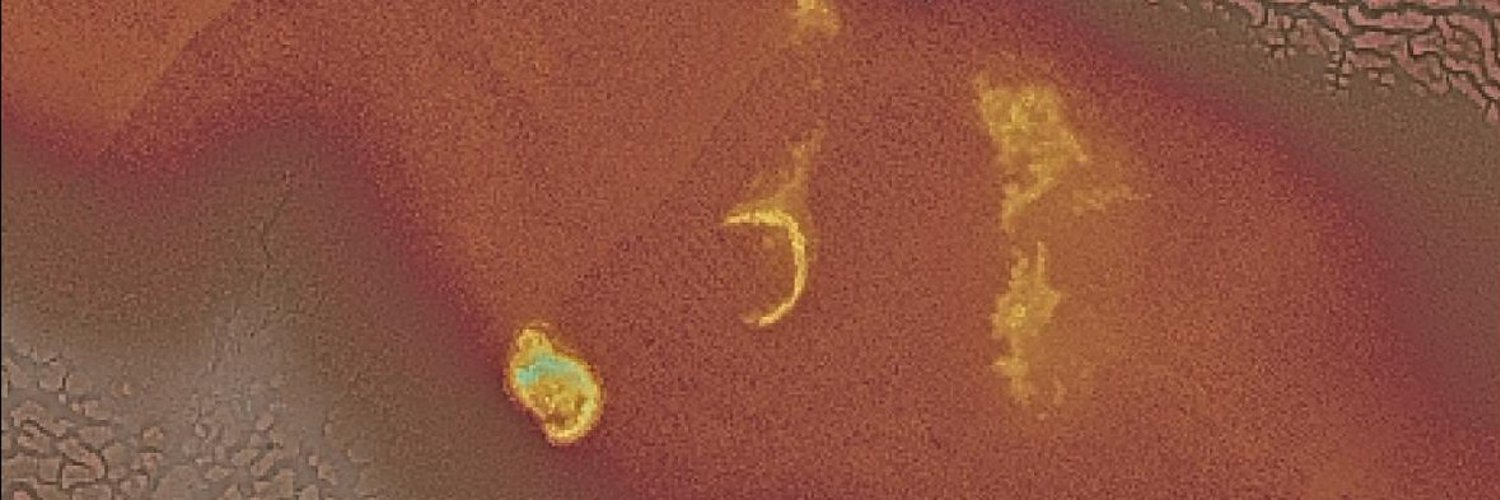
This project, which began with my Master's thesis, is focused on the development of automated image analysis methods for detecting pre-contact Native American mounds in the Southeastern United States from LiDAR datasets. LiDAR produce 3D points that can be used to see the ground’s surface, even in forested and heavily vegetated areas. We successfully detected over 100 mounds, many of which had not been recorded previously and which were located deep in forests. The earliest results of this project were published in Southeastern Archaeology and Journal of Archaeological Science: Reports. Central to the devised methods is a technique known as “object-based image analysis”, or OBIA. These methods attempt to detect whole objects in image data by looking for shape, size, and textural properties of an area, as well as underlying spectral/color data and matching these with a sample of known objects (for more information, see this article or listen to this podcast interview I gave on the subject).
Since 2018, this project has evolved to incorporate remotely sensed archaeological features to re-evaluate settlement patterns in this region. Using remotely sensed archaeological mounds in conjunction with existing datasets, my colleagues and I are using and spatial modeling methods to investigate the reason for shell ring placement in modern day Beaufort County, South Carolina. Shell rings are one specific type of mound architecture that is present throughout the American Southeast, but until recently only about 50 rings were recorded. My work using LiDAR and OBIA revealed many additional ring-like features, several of which have been confirmed as archaeological shell rings, to date. In continuing work, we are improving our original OBIA algorithm using a new method for automated object detection known as convolutional neural network analysis (or CNN) for evaluating mound building practices throughout the American Southeast.
Using CNNs, ongoing work is evaluating the spatial extent of shell ring building practices in this region. The results of this work were published in Journal of Archaeological Science. The future of this project will continue to examine Archaic settlement patterns along the entire Eastern coastline of the United States and investigate their relationship with climate events and environmental conditions.
Related Publications
Davis, D. S., Caspari, G., Lipo, C. P., & Sanger, M. C. (2021). Deep Learning Reveals Extent of Archaic Native American Shell-Ring Building Practices. Journal of Archaeological Science. 132, 105433. https://doi.org/10.1016/j.jas.2021.105433Davis, D. S., Caspari, G., Sanger, M. C., & Lipo, C. P. (2021). Using Deep Learning to detect rare archaeological features: A case from coastal South Carolina, USA. SEG Extended Technical Abstracts.
Davis, D. S., DiNapoli, R. J., Sanger, M. C., & Lipo, C. P. (2020). The integration of lidar and legacy datasets provides improved explanations for the spatial patterning of shell rings in the American Southeast. Advances in Archaeological Practice, 8(4), 361-375. https://doi.org/10.1017/aap.2020.18
Davis, D. S., Lipo, C. P., & Sanger, M. C. (2019). A comparison of automated object extraction methods for mound and shell-ring identification in coastal South Carolina. Journal of Archaeological Science: Reports, 23, 166–177. https://doi.org/10.1016/j.jasrep.2018.10.035
Davis, D. S., Sanger, M. C., & Lipo, C. P. (2019). Automated mound detection using lidar and object-based image analysis in Beaufort County, South Carolina. Southeastern Archaeology, 38(1), 23–37. https://doi.org/10.1080/0734578X.2018.1482186
Davis, D. S. (2019). Object-based image analysis: A review of developments and future directions of automated feature detection in landscape archaeology. Archaeological Prospection, 26(2), 155–163. https://doi.org/10.1002/arp.1730